Press Releases

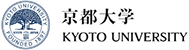
JAMSTEC
KYOTO UNIVERSITY
A New Method Using Image Classification AI to Predict Potential Natural Vegetation
1. Key Points
- ◆
- A new method using image classification AI to predict potential natural vegetation corresponding to climate (monthly precipitation and monthly mean air temperature of a year) was developed. This method can estimate potential natural vegetation with ease and precision compared with pre-existing methods.
- ◆
- The trained model accurately simulated a global map of current distributions of potential natural vegetation. Then, the trained model was applied to climate scenarios toward the end of the 21st century, predicting a significant shift in global biome distribution under rapid warming trends.
- ◆
- This method could also be applied to building empirical models of other climate-driven phenomena such as cropping systems and the spread of vector-borne diseases.
2. Overview
Scientific Researcher Hisashi SATO of the Institute of Arctic Climate and Environment Research (IACE), Research Institute for Global Change (RIGC), Japan Agency for Marine-Earth Science and Technology (JAMSTEC), and Assoc. Prof. Takeshi ISE of Kyoto University’s Field Science Education and Research Center jointly developed a new method using an image classification AI (*1) to estimate potential natural vegetation corresponding to given climates (*2).
This is the first application of image classification AI to reconstruct potential natural vegetation, and it offers a convenient and practical means to predict changes in vegetation distribution accompanying climate change. In this method, the image classification AI is trained to extract features of various types of potential natural vegetation based on seasonal change patterns represented in images. The trained AI was able to reproduce the current distribution of potential natural vegetation with better precision than pre-existing methods. Then, the trained AI was applied to climatic scenarios at the end of the 21st century, predicting a significant shift in global vegetation distributions such as (1) decreased forest density in the southern part of the Amazon basin, (2) expansion of temperate forests on the North American and Eurasian continents, and (3) a shift in the distribution of boreal forests towards higher latitudes and altitudes. Although these trends are qualitatively consistent with previous research works, improvements in quantitative reliability are expected.
Since this method is simply an application of image classification AI, it demands much less technical skill and computer resources. Therefore, this method could also be applied to building empirical models of other climate-driven phenomena such as cropping systems and the spread of vector-borne diseases and hence has the potential to be a de facto standard for building empirical models across a range of research and application fields.
This study was published in Geoscientific Model Development on April 18 (Japan time).
- Research Institute for Global Change (RIGC), Japan Agency for Marine-Earth Science and Technology (JAMSTEC)
- Field Science Education and Research Center (FSERC), Kyoto University
This study was funded by the Ministry of Education, Culture, Sports, Science and Technology’s Arctic Challenge for Sustainability II (ArCS II) (JPMXD1420318865) and the Japan Society for the Promotion of Science’s Grants-in-Aid for Scientific Research (18H03357).
【Supplemental Information】
- *1
- Image classification AI:
In this study, we used LeNet, one of the earliest image classification AIs. LeNet was originally developed for the purpose of handwritten digit recognition and has a relatively simple architecture (such as having relatively few so-called “hidden layers”). Therefore, it is well suited for the task of classifying relatively simple images into ten or so categories.
- *2
- Potential natural vegetation:
This term refers to a type of vegetation that ultimately stabilizes under a given set of environmental conditions (such as climate, geology, terrain, and latitude) in a situation where artificial impact is negligible. The method for classifying vegetation into different types varies depending on purpose and geographical range; in this study, we used the ISLSCP2 Potential Natural Vegetation Cover which classifies global vegetation into 15 types.
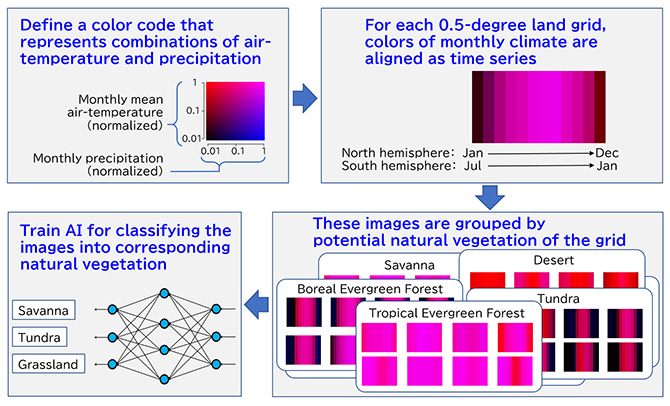
Fig.1 Overview of the method
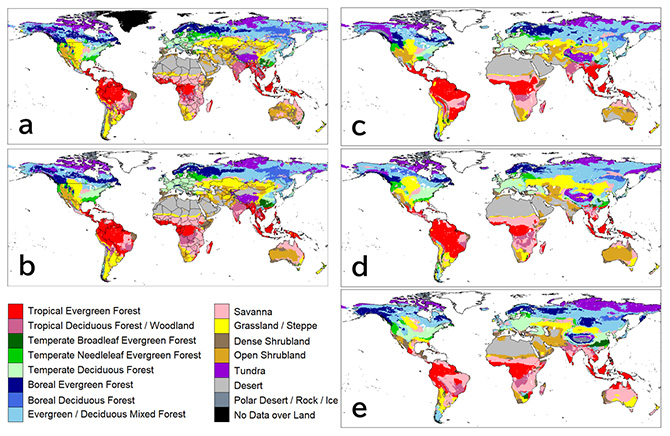
Fig.2 Distributions of potential natural vegetation
“a” is the observation-derived data (ISLSCP2) we used for training. “b” to “e” are distributions obtained by inputting average climates from 1971 to 1980 derived from various climate data sets into the trained model (b: CRU TS4.00, c: NCEP/NCAR Reanalysis data set, d: Had2GEM-ES, e: MIROC-ESM). CRU TS4.00 was used to train the classification of potential natural vegetation. Therefore, the degree to which “a” and “b” match indicates the learning efficiency of the image classification AI. “c” to “e,” which were obtained by inputting climate data sets that were not used for training, were computed to verify the generality of the model (the ability of the model to yield valid outputs when using different climate data sets). None of the model outputs “b” to “e” showed major discrepancies with the observation-derived data “a.”
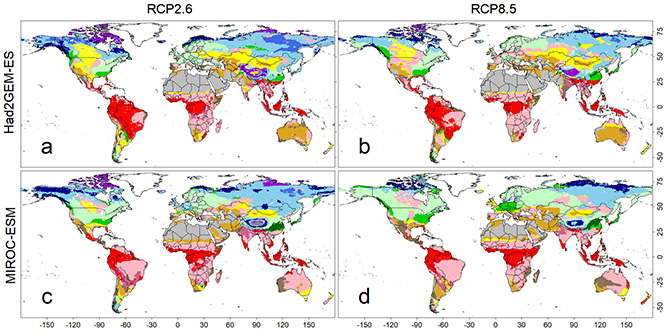
Fig.3 Predicted maps of potential natural vegetation under climatic scenarios from 2091 to 2100. Four sets of forecasted climatic conditions were derived from combinations of two climate models (i.e., Had2GEM-ES and MIROC-ESM) and two RCP scenarios (i.e., RCP2.6 and RCP8.5). Color definitions are available in Fig. 2.
Contacts
- (For this study)
- Hisashi Sato, Researcher, Research Institute for Global Change (RIGC), Institute of Arctic Climate and Environment Research(IACE), Arctic Geochemical Cycle Research Group, JAMSTEC
- (For press release)
- Press Office, Marine Science and Technology Strategy Department, JAMSTEC